PROBABILISTIC MATRIX FACTORIZATION CONTENT BASED RECOMMENDATION
More specifically the group and paper content information are integrated into the probabilistic matrix factorization model to enhance the accuracy of. Utility-based repair of inconsistent requirements 2009 Felfernig A Mairitsch M Mandl M et al.
Information Free Full Text An Extended Tag Induced Matrix Factorization Technique For Recommender Systems Html
A Probabilistic Matrix Factorization Recommendation Method Based on Deep Learning Xiaoyue Gong and Xiaojun Huang-Matrix factorization recommendation algorithms based on knowledge map representation learning 1 Xuejian Huang Lu Min Gensheng Wang et al-This content was downloaded from IP address 1575539100 on 13022022 at 0557.
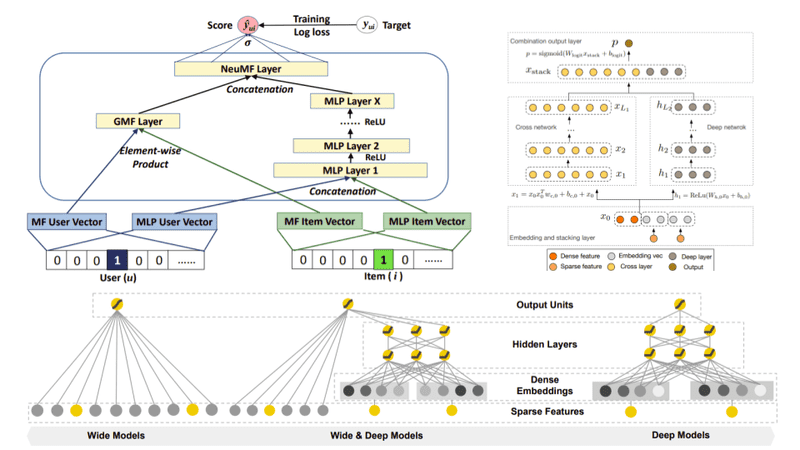
. But optimizing the objective function in conventional matrix factorization based recommendation methods which is the sum-of-square of factorization errors with regularization terms does not ensure that the obtained recommendation results are consistent with the preference orders of the users. For building recommendation systems probabilistic matrix factorization PMF is a prevailing method that performs well on large and sparse datasets. Embedding-based news recommendation for millions of users - 2017.
Both the ratings and textual documents are used in CTR. In this paper we describe approach of our SemWex1 group to the ESWC 2014 RecSys Challenge. Literature review of the advances of deep learning-based recommender system.
With the advent of social in uence social recommender sys-tems have become an active research topic for making recommendations based on the ratings of the users that have close social relations with the given user. Some of the social factors have been used in RS but have not been fully considered. Flexible Online Learning at Your Own Pace.
All other documents are new to the system. Wide Deep Learning for Recommender Systems - 2016. Invest 2-3 Hours A Week Advance Your Career.
This model regards the user preference matrix as a product of two lower-rank user and item matrices and adopts a probabilistic linear setting with Gaussian observation noise. Deep Learning Based Recommender System. Up to 10 cash back PMF.
In this paper three social factors personal interest interpersonal interest similarity and interpersonal influence fuse into a unified personalized recommendation model based on. Bayesian Probabilistic Matrix Factorization using Markov Chain Monte Carlo 2008R Salakhutdinov et al. Our method is based on using an adaptation of Content.
Collaborative topic regression is a recommendation algorithm which combines PMF and the latent Dirichlet allocation. The underlying assumption is that a users taste is. Up to 10 cash back In order to effectively solve the problem of new items and obviously improve the accuracy of the recommended results we proposed a collaborative recommendation algorithm based on improved probabilistic latent semantic model in this paper which introduces popularity factor into probabilistic latent semantic analysis to derive.
The rapid development of location-based social networks LBSNs has. This probabilistic model is equivalent to. This dot product calculation is derived from matrix factorization.
Probability Graph Model and Byesian Inference. We define in-matrix docu-ments as those that have been rated by at least one user in the recommendation system. A cold-start recommendation of a new document is based entirely on its content.
Content-based recommendations with Poisson factorization Prem Gopalan Laurent Charlin David M. In-matrix documents and out-matrix or cold-start documents to users. Coupled Group-based Matrix Factorization for Recommender System.
Modeling the topic modelModeling the geographical correlationsModeling the social correlationsModeling the categorical correlationsWe integrate the textual geographical social categorical and popularity information into probabilistic matrix factorization model for POI recommendation. Probabilistic matrix factorization is a basic method of rating prediction which uses the rating scores in the form of probability. App recommender system for Google Play with a wide and deep model.
Therefore a group-oriented paper recommendation method based on probabilistic matrix factorization and evidential reasoning GPMF_ER is proposed in this article to tackle these problems. Ad Build your Career in Data Science Web Development Marketing More. We introduce Poisson Matrix Factorization with Content and Social trust information PoissonMF-CS a latent variable prob-abilistic model for recommender systems with the objective of jointly modeling social trust item content and users preference using Poisson matrix factorization framework.
Blei Department of Computer Science Princeton University Princeton NJ 08540 fpgopalanlcharlinbleigcsprincetonedu Abstract We develop collaborative topic Poisson factorization CTPF a generative model of articles and reader preferences. A Survey and New Perspectives - 2019. To address this problem in this paper we improve the model of probabilistic matrix.
All current recommendation algorithms when modeling useritem interactions basically use dot product.
An Introduction To Recommendation Systems An Overview Of Machine And Deep Learning Architectures Ai Summer
Applied Sciences Free Full Text Recommendation System Using Autoencoders Html
Recommendation Systems Papers With Code
Tutorial Implementing Your Own Recommender Systems In Python By Cambridge Spark Cambridge Spark
Github Fuhailin Probabilistic Matrix Factorization Python Implementation Of Probabilistic Matrix Factorization Pmf Algorithm For Building A Recommendation System Using Movielens Ml 100k Grouplens Dataset
Movie Recommendation Systems Are Becoming Increasingly Important In Today S Extremely Busy Machine Learning Deep Learning Machine Learning Course Data Science
Introduction To Latent Matrix Factorization Recommender Systems By Tumas Rackaitis Towards Data Science
Information Free Full Text An Extended Tag Induced Matrix Factorization Technique For Recommender Systems Html
0 Response to "PROBABILISTIC MATRIX FACTORIZATION CONTENT BASED RECOMMENDATION"
Post a Comment